SBD Explores: The Secret Behind ChatGPT and its Implication to the Automotive Industry
- James Springthorpe
- Sep 3, 2023
- 4 min read
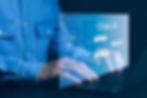
Large Language Models (LLMs), like the one powering ChatGPT, have shifted the paradigm of AI development with their ability to understand context and generate nuanced responses. Their application in the automotive industry could significantly enhance customer experience, assist in technical diagnostics, and contribute to autonomous vehicle development.
However, challenges & limitations need to be addressed before bringing this powerful technology into business operation. Such challenges and limitations include data and computing resource requirements, potential misinformation, privacy and data security.
What is happening?
There has been a paradigm shift in AI development within the past 6 months. LLMs have been established and look to achieve a massive leap in AI growth. Since this advancement, we are seeing many tech companies compete to develop more reliable, accurate, and intelligent systems.
There are numerous new AI powered applications released in the past months. Most of them utilize mainstream LLMs like GPT-3, GPT-4, PaLM and LLaMA.
LLMs are expensive. Training and fine tuning an LLM will cost millions of dollars for computing resources. This is excluding the cost of AI talents, data, and other infrastructures.
OPENAI is leading with support from Microsoft who provide computing resources and funding.
Some players, such as OPENAI, Google, and Microsoft, choose a “close-source” strategy for various reasons.
Meta AI is focused on developing new LLMs including Imagebind, SAM, MMS, and the famous LLaMA and decided go with an open-source approach.
Huggingface is the largest open-source AI community.

Why does it matter?
The new AI wave will impact many business processes in most industries. Automotive is no exception. SBD has identified 10 potential AI use cases and categorized them against four personal mobility outcomes.
LLM excels in multiple forms of language understanding, spanning human languages and programming languages.
Its superior capabilities compared to previous Deep Learning models include:
1. Proficiency in understanding and operating in various languages.
2. Ability to comprehend and retain common knowledge.
3. Proficiency in reasoning, a.k.a. the Chain of Thought (COT).
We are currently in the initial stages of advanced AI development, and the future trajectory of AI is fraught with uncertainty around how fast it will continue to develop and what legislation will arise to control/monitor AI.
There are also ongoing safety and privacy concerns that need to be addressed, such as potential misuse of input data for AI training.

Where next?
The capabilities of the newest LLMs exceed other current AI models, yet they are challenging to construct and costly to maintain. OEMs must thoroughly comprehend the implications of LLMs on various business operations before formulating a solid AI strategy and devising an actionable plan to enter the game. OEMs who are first to AI integration will have key advantages.
LLMs can greatly enhance software development efficiency/quality, allowing OEMs to swiftly meet customer demands.
LLMs are not a universal solution, certain AI models still excel in specific use cases. Hence, OEMs must integrate various models into a single platform, necessitating AI middleware for long-term management.
Some existing generative AI applications, such as ChatGPT, use prompts and inputs as training data, which will lead to privacy issue or data leakage. A clear policy needs to be in place before employees start to use AI for work.
Multi-modal is maturing. Despite AI's rapid yet early-stage progress towards Artificial Generative Intelligence (AGI), starting small, early projects can equip OEMs with crucial experience and understanding to harness AI's potential.
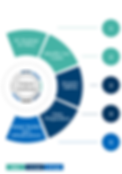
OEMs must devise a well-rounded AI strategy and policy to harness AI's potential for business growth, ensuring it remains secure and manageable.
Identify and select use cases that can address business challenges.
Acquire AI talents including data scientists and AI developers before they become even more expensive.
Prepare datasets required for LLM training & tuning. Make sure they are clean and in good quality.
Select the appropriate model for each application. With AI infrastructure being costly and scarce, OEMs must strategically choose their sourcing, considering productivity, finance, and data security.
What to watch out for?
Advanced AI development has accelerated in recent months, and the current competition is around building the most powerful LLM as it has shown the “Sparks of AGI".
LLM competition today is still a game of a few large tech giants who have sufficient funds, AI talents, and data.
Most leading LLMs today are closed-source and only Meta is taking an open-source approach. In the meantime, more opensource LLMs are developing rapidly.
There are three options for OEMs to have their own foundation model:
1. In-House build from scratch
2. Train from open-source model
3. Use AIaaS providers
More tools will be available in the next 1~2 years for enterprises to train or tune their foundational model at lower cost.
To fully embed an LLM into business applications, there will be another middleware layer required after the foundation model is trained.
AI infrastructure needs to be well planned technically and financially before loading LLM into production.

How should you react?
Understand
Start by understanding the capability and limitations of different LLMs and learn how to better utilize the power using prompt engineering.
Assess
Evaluate the potential use cases within the automotive industry and map them into your own business operations. Keeping your eyes on ethical consideration including bias and privacy.
Prepare
Define AI strategy and plan for key resources of building your own foundational model, including data, model, talents, and infrastructures.
Interested in finding out more?
Most of our work is helping clients go deeper into new challenges and opportunities through custom projects. If you would like to discuss recent projects we've completed relating to Artificial Intelligence and Large Language Models, contact us today!
Also, be sure to view our related content: